Google AI围棋已过九段!碾压欧洲冠军
来源:百度文库 编辑:超级军网 时间:2024/04/27 15:47:28
http://news.mydrivers.com/1/468/468596.htm
上周,Google AI在围棋上以战胜了5比0欧洲冠军樊麾的消息引起极大关注,而更为大家津津乐道的,是Google还向韩国围棋九段高手李世石公开发起挑战,要在下个月进行AI与围棋高手的较量。作为围棋界最高水平的代表,如果李世石输了,代表的是人类输了。
Google使用的人工智能软件是AlphaGo,由Google去年收购的人工智能公司DeepMind研发。
关于AlphaGo与李世石之间的这场较量,外界有着不同的看法,但总体而言,觉得赢得可能性低的人会更多些,因为樊麾仅为二段棋手,二段棋手与九段棋手之间,差得可不是一星半点。
而在围棋界,有人觉得AlphaGo之前的比赛并没有太多的说服力。根据Google公布的数据,AlphaGo进行的500局比赛中,只有与樊麾对弈的5局是有人类棋手参与的,其他495局是与其他围棋程序进行的。虽然AlphaGo的战绩可以用全胜来形容,不过缺乏与人类棋手的实战经验。
不过,近日在高手云集的弈城围棋网上,出现了疑似AlphaGo的身影,而且从它的AlphaGo对战数量和战绩上看,它已经和人类进行了大量的交手,甚至最好的成绩达到过9D(段)。
弈城工作人员在弈城网的众多高手ID中,发现了一个注册为“英国”的、名为“deepmind”的账号,这个账号曾在弈城9D上站了4个多月,于上周日被打回了8D。
当然,目前并不能确定这个“deepmind”就是Google研发团队Deep Mind的测试账号,但仔细分析一下这个账号在弈城的对弈记录,也颇有意思。

注册为“deepmind”的英国账号
deepmind目前在弈城的总战绩为465战276胜186负3和。
deepmind的第一盘对局是2014年4月2日,当时它注册的段位是5D,此后在5D上取得了17连胜,直到4月13日输给了woocl,跳升7D未果,段位升为6D。
之后,4天之内deepmind在6D上15胜3败,于4月17日升为7D。紧接着,5天之内deepmind在7D上下了27盘棋,19胜8负,于4月22日升为8D。

deepmind部分对局记录
之后deepmind的进阶之路开始出现不顺利,在90多盘输赢不定后,在8月31日被降回了7D。两周后的9月14日,deepmind再度升回8D。
之后的一年时间里,deepmind在7D和8D之间又经过了三次升降,终于在2015年9月16日升上了弈城9D。
直到2015年12月3日,deepmind在弈城网上第一次击败职业棋手(“P”号为职业棋手),之后又相继战胜了两个“P号”。
而deepmind最受围棋爱好者关注的一场对弈发生在今年1月23日,对手是日本著名ID“3586”,关于此号的真身一直有诸多猜测,甚至有棋迷认为是日本第一人井山裕太。

deepmind执白67手中盘负于3586
在这场读一中,仅67手即中盘脆败。执黑的deepmind大龙被屠而终局,其时右边盘尚空空如也,这样的战况在deepmind的对局中屡见不鲜,它好像对布局不大感兴趣,更加偏爱局部作战。就在输给3586的第二天,deepmind又被降回8D。
deepmind的ID被网友扒出后,引起了10年来一直研究人工智能围棋的韩国六段棋手金灿佑的注意。
金灿佑仔细查看了deepmind的棋谱,提出了两点看法:第一,普通人的棋力不太可能在一年多的时间里从弈城5D、6D迅速提高到弈城9D,但Google的AlphaGo是有这个可能的。
第二,deepmind在5D时的棋谱中,很多手段都明显有以前人工智能围棋软件的痕迹,很像是软件的下法。
所以综合这两点来看,deepmind很有可能是GoogleDeepMind团队为测试AlphaGo而注册的ID。
如此看来,deepmind为AlphaGo的ID可能性很大,而AlphaGo的围棋水平其实早就超出了我们的预期。虽然与九段棋手有一段距离,但赢李世石的几率已经大大提升了。

韩国九段棋手李世石
http://news.mydrivers.com/1/468/468596.htm
上周,Google AI在围棋上以战胜了5比0欧洲冠军樊麾的消息引起极大关注,而更为大家津津乐道的,是Google还向韩国围棋九段高手李世石公开发起挑战,要在下个月进行AI与围棋高手的较量。作为围棋界最高水平的代表,如果李世石输了,代表的是人类输了。
Google使用的人工智能软件是AlphaGo,由Google去年收购的人工智能公司DeepMind研发。
关于AlphaGo与李世石之间的这场较量,外界有着不同的看法,但总体而言,觉得赢得可能性低的人会更多些,因为樊麾仅为二段棋手,二段棋手与九段棋手之间,差得可不是一星半点。
而在围棋界,有人觉得AlphaGo之前的比赛并没有太多的说服力。根据Google公布的数据,AlphaGo进行的500局比赛中,只有与樊麾对弈的5局是有人类棋手参与的,其他495局是与其他围棋程序进行的。虽然AlphaGo的战绩可以用全胜来形容,不过缺乏与人类棋手的实战经验。
不过,近日在高手云集的弈城围棋网上,出现了疑似AlphaGo的身影,而且从它的AlphaGo对战数量和战绩上看,它已经和人类进行了大量的交手,甚至最好的成绩达到过9D(段)。
弈城工作人员在弈城网的众多高手ID中,发现了一个注册为“英国”的、名为“deepmind”的账号,这个账号曾在弈城9D上站了4个多月,于上周日被打回了8D。
当然,目前并不能确定这个“deepmind”就是Google研发团队Deep Mind的测试账号,但仔细分析一下这个账号在弈城的对弈记录,也颇有意思。

注册为“deepmind”的英国账号
deepmind目前在弈城的总战绩为465战276胜186负3和。
deepmind的第一盘对局是2014年4月2日,当时它注册的段位是5D,此后在5D上取得了17连胜,直到4月13日输给了woocl,跳升7D未果,段位升为6D。
之后,4天之内deepmind在6D上15胜3败,于4月17日升为7D。紧接着,5天之内deepmind在7D上下了27盘棋,19胜8负,于4月22日升为8D。

deepmind部分对局记录
之后deepmind的进阶之路开始出现不顺利,在90多盘输赢不定后,在8月31日被降回了7D。两周后的9月14日,deepmind再度升回8D。
之后的一年时间里,deepmind在7D和8D之间又经过了三次升降,终于在2015年9月16日升上了弈城9D。
直到2015年12月3日,deepmind在弈城网上第一次击败职业棋手(“P”号为职业棋手),之后又相继战胜了两个“P号”。
而deepmind最受围棋爱好者关注的一场对弈发生在今年1月23日,对手是日本著名ID“3586”,关于此号的真身一直有诸多猜测,甚至有棋迷认为是日本第一人井山裕太。

deepmind执白67手中盘负于3586
在这场读一中,仅67手即中盘脆败。执黑的deepmind大龙被屠而终局,其时右边盘尚空空如也,这样的战况在deepmind的对局中屡见不鲜,它好像对布局不大感兴趣,更加偏爱局部作战。就在输给3586的第二天,deepmind又被降回8D。
deepmind的ID被网友扒出后,引起了10年来一直研究人工智能围棋的韩国六段棋手金灿佑的注意。
金灿佑仔细查看了deepmind的棋谱,提出了两点看法:第一,普通人的棋力不太可能在一年多的时间里从弈城5D、6D迅速提高到弈城9D,但Google的AlphaGo是有这个可能的。
第二,deepmind在5D时的棋谱中,很多手段都明显有以前人工智能围棋软件的痕迹,很像是软件的下法。
所以综合这两点来看,deepmind很有可能是GoogleDeepMind团队为测试AlphaGo而注册的ID。
如此看来,deepmind为AlphaGo的ID可能性很大,而AlphaGo的围棋水平其实早就超出了我们的预期。虽然与九段棋手有一段距离,但赢李世石的几率已经大大提升了。

韩国九段棋手李世石
上周,Google AI在围棋上以战胜了5比0欧洲冠军樊麾的消息引起极大关注,而更为大家津津乐道的,是Google还向韩国围棋九段高手李世石公开发起挑战,要在下个月进行AI与围棋高手的较量。作为围棋界最高水平的代表,如果李世石输了,代表的是人类输了。
Google使用的人工智能软件是AlphaGo,由Google去年收购的人工智能公司DeepMind研发。
关于AlphaGo与李世石之间的这场较量,外界有着不同的看法,但总体而言,觉得赢得可能性低的人会更多些,因为樊麾仅为二段棋手,二段棋手与九段棋手之间,差得可不是一星半点。
而在围棋界,有人觉得AlphaGo之前的比赛并没有太多的说服力。根据Google公布的数据,AlphaGo进行的500局比赛中,只有与樊麾对弈的5局是有人类棋手参与的,其他495局是与其他围棋程序进行的。虽然AlphaGo的战绩可以用全胜来形容,不过缺乏与人类棋手的实战经验。
不过,近日在高手云集的弈城围棋网上,出现了疑似AlphaGo的身影,而且从它的AlphaGo对战数量和战绩上看,它已经和人类进行了大量的交手,甚至最好的成绩达到过9D(段)。
弈城工作人员在弈城网的众多高手ID中,发现了一个注册为“英国”的、名为“deepmind”的账号,这个账号曾在弈城9D上站了4个多月,于上周日被打回了8D。
当然,目前并不能确定这个“deepmind”就是Google研发团队Deep Mind的测试账号,但仔细分析一下这个账号在弈城的对弈记录,也颇有意思。

注册为“deepmind”的英国账号
deepmind目前在弈城的总战绩为465战276胜186负3和。
deepmind的第一盘对局是2014年4月2日,当时它注册的段位是5D,此后在5D上取得了17连胜,直到4月13日输给了woocl,跳升7D未果,段位升为6D。
之后,4天之内deepmind在6D上15胜3败,于4月17日升为7D。紧接着,5天之内deepmind在7D上下了27盘棋,19胜8负,于4月22日升为8D。

deepmind部分对局记录
之后deepmind的进阶之路开始出现不顺利,在90多盘输赢不定后,在8月31日被降回了7D。两周后的9月14日,deepmind再度升回8D。
之后的一年时间里,deepmind在7D和8D之间又经过了三次升降,终于在2015年9月16日升上了弈城9D。
直到2015年12月3日,deepmind在弈城网上第一次击败职业棋手(“P”号为职业棋手),之后又相继战胜了两个“P号”。
而deepmind最受围棋爱好者关注的一场对弈发生在今年1月23日,对手是日本著名ID“3586”,关于此号的真身一直有诸多猜测,甚至有棋迷认为是日本第一人井山裕太。

deepmind执白67手中盘负于3586
在这场读一中,仅67手即中盘脆败。执黑的deepmind大龙被屠而终局,其时右边盘尚空空如也,这样的战况在deepmind的对局中屡见不鲜,它好像对布局不大感兴趣,更加偏爱局部作战。就在输给3586的第二天,deepmind又被降回8D。
deepmind的ID被网友扒出后,引起了10年来一直研究人工智能围棋的韩国六段棋手金灿佑的注意。
金灿佑仔细查看了deepmind的棋谱,提出了两点看法:第一,普通人的棋力不太可能在一年多的时间里从弈城5D、6D迅速提高到弈城9D,但Google的AlphaGo是有这个可能的。
第二,deepmind在5D时的棋谱中,很多手段都明显有以前人工智能围棋软件的痕迹,很像是软件的下法。
所以综合这两点来看,deepmind很有可能是GoogleDeepMind团队为测试AlphaGo而注册的ID。
如此看来,deepmind为AlphaGo的ID可能性很大,而AlphaGo的围棋水平其实早就超出了我们的预期。虽然与九段棋手有一段距离,但赢李世石的几率已经大大提升了。

韩国九段棋手李世石
http://news.mydrivers.com/1/468/468596.htm
上周,Google AI在围棋上以战胜了5比0欧洲冠军樊麾的消息引起极大关注,而更为大家津津乐道的,是Google还向韩国围棋九段高手李世石公开发起挑战,要在下个月进行AI与围棋高手的较量。作为围棋界最高水平的代表,如果李世石输了,代表的是人类输了。
Google使用的人工智能软件是AlphaGo,由Google去年收购的人工智能公司DeepMind研发。
关于AlphaGo与李世石之间的这场较量,外界有着不同的看法,但总体而言,觉得赢得可能性低的人会更多些,因为樊麾仅为二段棋手,二段棋手与九段棋手之间,差得可不是一星半点。
而在围棋界,有人觉得AlphaGo之前的比赛并没有太多的说服力。根据Google公布的数据,AlphaGo进行的500局比赛中,只有与樊麾对弈的5局是有人类棋手参与的,其他495局是与其他围棋程序进行的。虽然AlphaGo的战绩可以用全胜来形容,不过缺乏与人类棋手的实战经验。
不过,近日在高手云集的弈城围棋网上,出现了疑似AlphaGo的身影,而且从它的AlphaGo对战数量和战绩上看,它已经和人类进行了大量的交手,甚至最好的成绩达到过9D(段)。
弈城工作人员在弈城网的众多高手ID中,发现了一个注册为“英国”的、名为“deepmind”的账号,这个账号曾在弈城9D上站了4个多月,于上周日被打回了8D。
当然,目前并不能确定这个“deepmind”就是Google研发团队Deep Mind的测试账号,但仔细分析一下这个账号在弈城的对弈记录,也颇有意思。

注册为“deepmind”的英国账号
deepmind目前在弈城的总战绩为465战276胜186负3和。
deepmind的第一盘对局是2014年4月2日,当时它注册的段位是5D,此后在5D上取得了17连胜,直到4月13日输给了woocl,跳升7D未果,段位升为6D。
之后,4天之内deepmind在6D上15胜3败,于4月17日升为7D。紧接着,5天之内deepmind在7D上下了27盘棋,19胜8负,于4月22日升为8D。

deepmind部分对局记录
之后deepmind的进阶之路开始出现不顺利,在90多盘输赢不定后,在8月31日被降回了7D。两周后的9月14日,deepmind再度升回8D。
之后的一年时间里,deepmind在7D和8D之间又经过了三次升降,终于在2015年9月16日升上了弈城9D。
直到2015年12月3日,deepmind在弈城网上第一次击败职业棋手(“P”号为职业棋手),之后又相继战胜了两个“P号”。
而deepmind最受围棋爱好者关注的一场对弈发生在今年1月23日,对手是日本著名ID“3586”,关于此号的真身一直有诸多猜测,甚至有棋迷认为是日本第一人井山裕太。

deepmind执白67手中盘负于3586
在这场读一中,仅67手即中盘脆败。执黑的deepmind大龙被屠而终局,其时右边盘尚空空如也,这样的战况在deepmind的对局中屡见不鲜,它好像对布局不大感兴趣,更加偏爱局部作战。就在输给3586的第二天,deepmind又被降回8D。
deepmind的ID被网友扒出后,引起了10年来一直研究人工智能围棋的韩国六段棋手金灿佑的注意。
金灿佑仔细查看了deepmind的棋谱,提出了两点看法:第一,普通人的棋力不太可能在一年多的时间里从弈城5D、6D迅速提高到弈城9D,但Google的AlphaGo是有这个可能的。
第二,deepmind在5D时的棋谱中,很多手段都明显有以前人工智能围棋软件的痕迹,很像是软件的下法。
所以综合这两点来看,deepmind很有可能是GoogleDeepMind团队为测试AlphaGo而注册的ID。
如此看来,deepmind为AlphaGo的ID可能性很大,而AlphaGo的围棋水平其实早就超出了我们的预期。虽然与九段棋手有一段距离,但赢李世石的几率已经大大提升了。

韩国九段棋手李世石
电脑什么时候能不依赖人发明一种棋类游戏才厉害,掷骰子的那种不算
吹~~~~~~
也有可能是脸书的软件dark forest?
人可能受环境和精力的影响,尤其是现代围棋比赛引入了读秒机制。比如老聂,擂台赛战绩辉煌,国际比赛当时还不是日本棋手的天下
真心不用李世石 随便来个围甲主力 就切掉了 而且机器是有弱点的 多下几盘 等那些职业发现了连业余都随便上 ps 那个欧洲的冠军早年是个职业 现在水平不如国内的强业余
fantacom 发表于 2016-2-4 12:05
真心不用李世石 随便来个围甲主力 就切掉了 而且机器是有弱点的 多下几盘 等那些职业发现了连业余都随便上 ...
说得好像你跟AlphaGo交过手似的。
真心不用李世石 随便来个围甲主力 就切掉了 而且机器是有弱点的 多下几盘 等那些职业发现了连业余都随便上 ...
说得好像你跟AlphaGo交过手似的。
还九段呢,呵呵,弈城的九段
说得好像你跟AlphaGo交过手似的。
你交过手 好吧
你交过手 好吧
fantacom 发表于 2016-2-4 22:22
你交过手 好吧
就算一个职业9段,也不敢保证5:0战胜一个职业2段。5:0是一个非常悬殊的比分好不好。
至于“早年是个职业,现在水平不如国内强业余”这种P话,只能呵呵。好歹人家是法国围棋队总教练,最近3年连续的欧洲冠军。虽然欧洲围棋水平一般,但还轮不到国内业余选手欺负。
而且AlphaGo是深度学习网络,可以总结弈局用来增长自己棋力,现在它每天自己对弈100万局,棋力增长迅速。
“等多下几盘”,呵呵去吧,多下几盘的时间,够Alpha升段了。
给AlphaGo5年时间,它能把李世石打得毛都不剩,走着瞧。
fantacom 发表于 2016-2-4 22:22
你交过手 好吧
就算一个职业9段,也不敢保证5:0战胜一个职业2段。5:0是一个非常悬殊的比分好不好。
至于“早年是个职业,现在水平不如国内强业余”这种P话,只能呵呵。好歹人家是法国围棋队总教练,最近3年连续的欧洲冠军。虽然欧洲围棋水平一般,但还轮不到国内业余选手欺负。
而且AlphaGo是深度学习网络,可以总结弈局用来增长自己棋力,现在它每天自己对弈100万局,棋力增长迅速。
“等多下几盘”,呵呵去吧,多下几盘的时间,够Alpha升段了。
给AlphaGo5年时间,它能把李世石打得毛都不剩,走着瞧。
大家都没看到重点,重点是李世石有什么资格代表围棋代表人类?西方就是这样潜移默化攻击中国的。
大家都没看到重点,重点是李世石有什么资格代表围棋代表人类?西方就是这样潜移默化攻击中国的。
这十年里,李世石的成绩很好!拿了最多的冠军,选他是合适的。
这十年里,李世石的成绩很好!拿了最多的冠军,选他是合适的。
不动大冥王 发表于 2016-2-5 13:29
这十年里,李世石的成绩很好!拿了最多的冠军,选他是合适的。
政治敏感性要加强,斗争无处不在
这十年里,李世石的成绩很好!拿了最多的冠军,选他是合适的。
政治敏感性要加强,斗争无处不在
就算一个职业9段,也不敢保证5:0战胜一个职业2段。5:0是一个非常悬殊的比分好不好。
至于“早年是 ...
能把李世石打得毛都不剩的话那就真的是秒杀全人类的棋手了
本次对决李世石能让几子还是人类艰难取胜?或者alphago艰难取胜?
至于“早年是 ...
能把李世石打得毛都不剩的话那就真的是秒杀全人类的棋手了
本次对决李世石能让几子还是人类艰难取胜?或者alphago艰难取胜?
这台电脑就像周伯通。一天内自己对弈N局,左右互搏,武功深不可测
导弹与捣蛋 发表于 2016-2-5 14:24
能把李世石打得毛都不剩的话那就真的是秒杀全人类的棋手了
本次对决李世石能让几子还是人类艰难取胜? ...
这次比赛李世石不让子,这么说吧,让子的话说明段位差着3-5段了。从之前的战绩看,电脑和李世石之剑的差距没有3-5段这么多。让几子?让5子的话,职业初段都能干掉李世石。
这次我看好李世石,艰难不艰难不好说,应该会赢。
但是我说他毛都不剩,是5年后。想国际象棋电脑第一次战胜顶尖人类选手,到顶尖人类最后一次战胜机器,只用了短短几年的时间。
能把李世石打得毛都不剩的话那就真的是秒杀全人类的棋手了
本次对决李世石能让几子还是人类艰难取胜? ...
这次比赛李世石不让子,这么说吧,让子的话说明段位差着3-5段了。从之前的战绩看,电脑和李世石之剑的差距没有3-5段这么多。让几子?让5子的话,职业初段都能干掉李世石。
这次我看好李世石,艰难不艰难不好说,应该会赢。
但是我说他毛都不剩,是5年后。想国际象棋电脑第一次战胜顶尖人类选手,到顶尖人类最后一次战胜机器,只用了短短几年的时间。
孙殿英1 发表于 2016-2-5 11:56
大家都没看到重点,重点是李世石有什么资格代表围棋代表人类?西方就是这样潜移默化攻击中国的。
无处吐槽,你阶级斗争的弦是不是绷得过紧了?不怕断了?
大家都没看到重点,重点是李世石有什么资格代表围棋代表人类?西方就是这样潜移默化攻击中国的。
无处吐槽,你阶级斗争的弦是不是绷得过紧了?不怕断了?
这十年里,李世石的成绩很好!拿了最多的冠军,选他是合适的。
他状态已经不行了。
他状态已经不行了。
下棋这种东西对电脑来说,并不是什么智商问题,都是设计程序的人太厉害了,电脑只负责运算,就像翻转棋,最强棋力的翻转棋程序电脑直接推算出往后的24步怎么走最好,从第一颗子开始就知道最后一颗子的结局了,和这样的程序下棋人类能赢?能赢才有鬼
jiasdmingzu 发表于 2016-2-6 23:10
下棋这种东西对电脑来说,并不是什么智商问题,都是设计程序的人太厉害了,电脑只负责运算,就像翻转棋,最 ...
你拿翻转棋跟围棋比运算量也是醉了。
不用别人,我做的翻转棋就足够秒杀一切人类选手了。
下棋这种东西对电脑来说,并不是什么智商问题,都是设计程序的人太厉害了,电脑只负责运算,就像翻转棋,最 ...
你拿翻转棋跟围棋比运算量也是醉了。
不用别人,我做的翻转棋就足够秒杀一切人类选手了。
你拿翻转棋跟围棋比运算量也是醉了。
不用别人,我做的翻转棋就足够秒杀一切人类选手了。
呵呵,说得好像有什么本质区别一样。。。发出来试试,还秒杀一切人类选手。最强棋力的软件也做不到秒杀,也可能平局
不用别人,我做的翻转棋就足够秒杀一切人类选手了。
呵呵,说得好像有什么本质区别一样。。。发出来试试,还秒杀一切人类选手。最强棋力的软件也做不到秒杀,也可能平局
这次alphago的胜率,一百成里有五成吧~
晚上的太阳 发表于 2016-2-4 12:22
还九段呢,呵呵,弈城的九段
这段数,呵呵
还九段呢,呵呵,弈城的九段
这段数,呵呵
孙殿英1 发表于 2016-2-5 13:50
政治敏感性要加强,斗争无处不在
这个妥妥的
政治敏感性要加强,斗争无处不在
这个妥妥的
burn 发表于 2016-2-5 14:50
这台电脑就像周伯通。一天内自己对弈N局,左右互搏,武功深不可测
这个相当不可测
这台电脑就像周伯通。一天内自己对弈N局,左右互搏,武功深不可测
这个相当不可测
谷歌软件,这个比较有意思
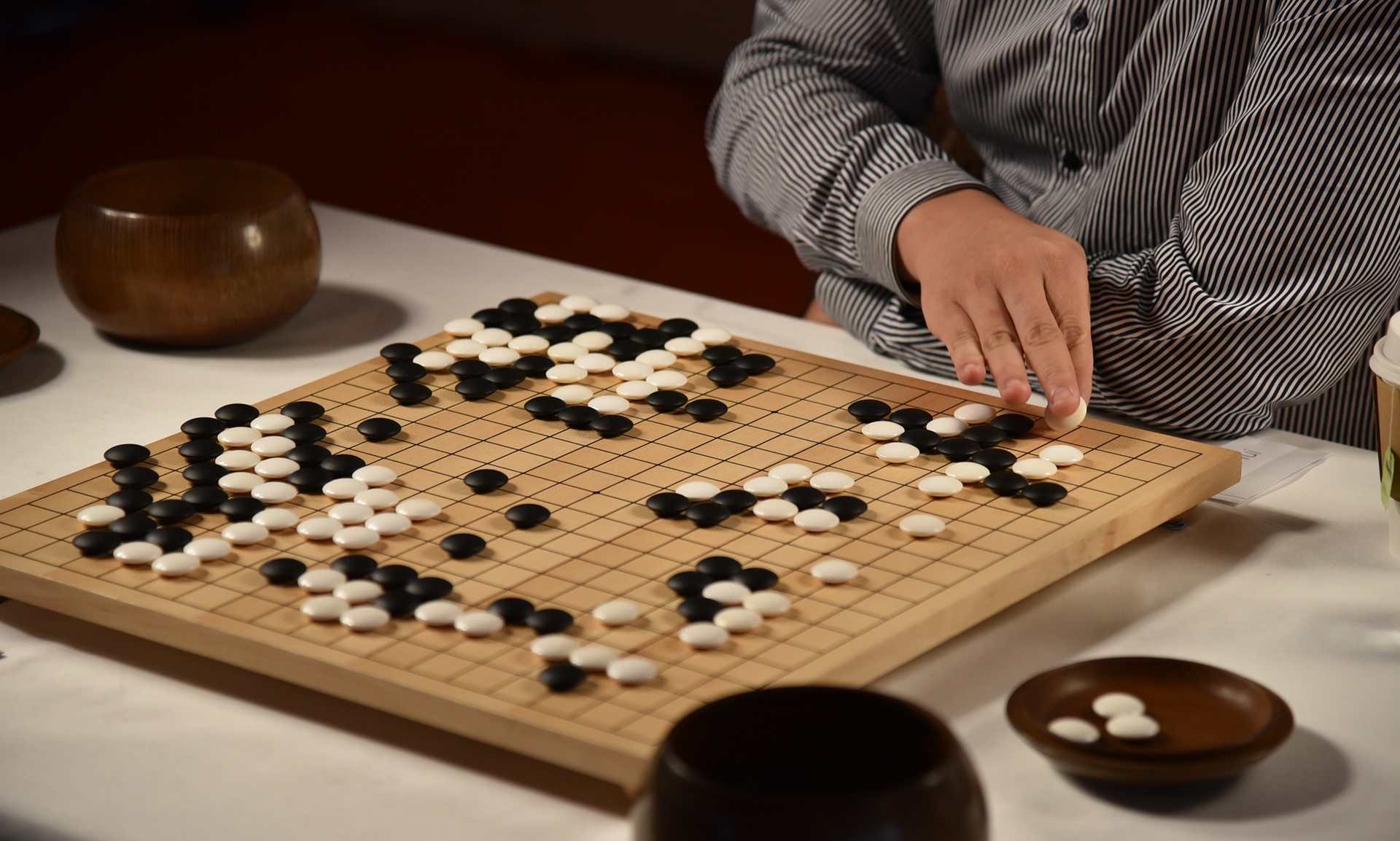
Fan Hui, three-time champion of the east Asian board game, lost to DeepMind’s program AlphaGo in five straight games.
Fan Hui makes a move against AlphaGo in DeepMind’s HQ in King’s Cross. Photograph: Google DeepMind
When Gary Kasparov lost to chess computer Deep Blue in 1997, IBM marked a milestone in the history of artificial intelligence. On Wednesday, in a research paper released in Nature, Google earned its own position in the history books, with the announcement that its subsidiary DeepMind has built a system capable of beating the best human players in the world at the east Asian board game Go.
Go, a game that involves placing black or white tiles on a 19x19 board and trying to remove your opponents’, is far more difficult for a computer to master than a game such as chess.
DeepMind’s software, AlphaGo, successfully beat the three-time European Go champion Fan Hui 5–0 in a series of games at the company’s headquarters in King’s Cross last October. Dr Tanguy Chouard, a senior editor at Nature who attended the matches as part of the review process, described the victory as “really chilling to watch”.
“It was one of the most exciting moments of my career,” he added. “But with the usual mixed feelings … in the quiet room downstairs, one couldn’t help but root for the poor human being beaten.”
It’s the first such victory for a computer program, and it came a decade before anyone expected it. As recently as 2014, Rémi Coulom, developer of the previous leading Go game AI, Crazy Stone, had predicted that it would take 10 more years for a machine to win against a top-rated human player without a handicap.
AlphaGo beat all expectations by approaching the challenge in a completely different way from previous software. Building on techniques DeepMind had employed in other feats of artificial intelligence, such as its system that could learn to play retro video games, AlphaGo used what the company calls “Deep Learning” to build up its own understanding of the game. It could then pick the moves it thought most likely to win.
When teaching a computer to play a game, the simplest method is to tell it to rank every possible move over the course of the game, from best to worst, and then instruct it to always pick the best move. That sort of strategy works for trivial games such as draughts and noughts and crosses, which have both been “solved” by computers that have fully examined every board state and worked out a way to play to at least a draw, no matter what the other player does.
However, for complex games such as Chess, the simple approach fails. Chess is just too big: in each turn there are approximately 35 legal moves, and a game lasts for around 80 turns. Enumerating every board position becomes computationally impossible very quickly, which is why it took so many years for IBM’s team to work out a way to beat Kasparov.
Go is bigger still. The definition of easy to learn, hard to master, it essentially has just two rules governing the core play, which involves two players alternately placing black and white tiles on a 19x19 board. The stones must be placed with at least one empty space next to it, or part of a group of stones of the same colour with at least one empty space, and if they lose their “liberty”, they are removed from the board.
While a game of chess might have 35 legal moves each turn, a game of Go has around 250 (including 361 legal starting positions alone); where Chess games last around 80 turns, Go games last 150. If Google had tried to solve the game in the same way noughts and crosses was solved, it would have had to examine and rank an obscene amount of possible positions: in the ballpark of 1,000,000,000,000,000,000,000,000,000,000,000,000,000,000,000,000,000,000,000,000,000,000,000,000,000,000,000,000,000,000,000,000,000,000,000,000,000,000,000,000,000,000,000,000,000,000,000,000,000,000,000,000,000,000,000,000,000 of them.
That renders an exhaustive search impossible, and even a selective search, of the style used by Deep Blue to defeat Kasparov, tricky to run efficiently.
Adding to the woes of those trying to master Go is the fact that, unlike chess, it’s very difficult to look at the board and mathematically determine who is winning. In chess, a player with their queen will probably beat a player whose queen has been taken, and so on: it’s possible to assign values to those pieces, and come up with a running score that roughly ranks each player’s prospects. In Go, by contrast, counters are rarely removed from the board, and there’s no simple mathematical way to determine who is in the stronger position until the game is very far progressed.
So AlphaGo focused on a very different strategy. As David Silver, DeepMind’s co-lead researcher on the project, puts it: “AlphaGo looks ahead by playing out the rest of the game in its imagination, many times over.” The program involves two neural networks, software that mimics the structure of the human brain to aggregate very simple decisions into complex choices, running in parallel.
One, the policy network, was trained by observing millions of boards of Go uploaded to an online archive. Using those observations, it built up a predictive model of where it expected the next piece to be played, given knowledge of the board and all previous positions, that could accurately guess the next move of an expert player 57% of the time (compared to a previous record of 44.4% from other groups).
This “supervised learning” was then backed up by a bout of “reinforcement learning”: the network was set to play against itself, learning from its victories and losses as it carried out more than 1m individual games over the course of a day.
The policy network was capable of predicting the probability that any given move would be played as next, but the system also needed a second filter to help it select which of those moves was the best. That network, the “value network”, predicts the winner of the game given each particular board state.
Building AlphaGo isn’t just important as a feather in DeepMind’s cap. The company argues that perfecting deep learning techniques such as this are crucial for its future work. Demis Hassabis, DeepMind’s founder, says that “ultimately we want to apply these techniques in important real-world problems, from medical diagnostics to climate modelling”.
For now, the DeepMind team is focused on one final goal on the Go board: a match against Lee Se-dol, the world champion. Lee says that “regardless of the result, it will be a meaningful event in the baduk (the Korean name for Go) history. I heard Google DeepMind’s AI is surprisingly strong and getting stronger, but I am confident that I can win at least this time.”
jiasdmingzu 发表于 2016-2-8 14:33
呵呵,说得好像有什么本质区别一样。。。发出来试试,还秒杀一切人类选手。最强棋力的软件也做不到秒杀, ...
扯淡,你当是3X3的翻转棋呢?还平局。平局?做大头梦去吧。
你懂毛线,还有大言不惭什么本质区别。说秒杀都是看得起你,现在的翻转棋可以从第一步开始就做终局运算,而不是仅评估局面。
而围棋,包括国际象棋,从来都不是靠做终局运算的,你懂么?
其实不止是翻转棋了,除了围棋,现在所有棋类电脑都可以轻松战胜人类选手。
呵呵,说得好像有什么本质区别一样。。。发出来试试,还秒杀一切人类选手。最强棋力的软件也做不到秒杀, ...
扯淡,你当是3X3的翻转棋呢?还平局。平局?做大头梦去吧。
你懂毛线,还有大言不惭什么本质区别。说秒杀都是看得起你,现在的翻转棋可以从第一步开始就做终局运算,而不是仅评估局面。
而围棋,包括国际象棋,从来都不是靠做终局运算的,你懂么?
其实不止是翻转棋了,除了围棋,现在所有棋类电脑都可以轻松战胜人类选手。
就算一个职业9段,也不敢保证5:0战胜一个职业2段。5:0是一个非常悬殊的比分好不好。
至于“早年是 ...
就有着瞧 法国队总教练 我还国足教头呢 让他和国内的马天放下下 入了职业初段不在职业圈混的 下不过业余很正常 职业九段下不赢二段 那要看啥九段啥二段 等级分才是王道 段位已经意义不大了 说了不再国内混职业圈 棋力下降的厉害 去欧洲的都是去扶贫的 就是古力去了欧洲 过两年也不入流 要保持水平只有在激烈的国内比赛一直竞争
至于“早年是 ...
就有着瞧 法国队总教练 我还国足教头呢 让他和国内的马天放下下 入了职业初段不在职业圈混的 下不过业余很正常 职业九段下不赢二段 那要看啥九段啥二段 等级分才是王道 段位已经意义不大了 说了不再国内混职业圈 棋力下降的厉害 去欧洲的都是去扶贫的 就是古力去了欧洲 过两年也不入流 要保持水平只有在激烈的国内比赛一直竞争
就有着瞧 法国队总教练 我还国足教头呢 让他和国内的马天放下下 入了职业初段不在职业圈混的 下不过业余 ...
欧洲围棋的水平 就国足在世界上的地位 拿个欧洲冠军做噱头 就说明这玩意没什么厉害的 别李世石了 把国内那些刚入段的小孩拿出来练练 我就不提韩国那些怪物初段了
欧洲围棋的水平 就国足在世界上的地位 拿个欧洲冠军做噱头 就说明这玩意没什么厉害的 别李世石了 把国内那些刚入段的小孩拿出来练练 我就不提韩国那些怪物初段了
进步好快呀!记得电脑刚击败国际象棋时,媒体还说要击败围棋至少还要60年。
今天特意去看了看这个id的棋。输赢都很惨烈,赢的棋大部分都是屠龙获胜。但输的棋也有很多脆败的。
打谱的感觉,很像是电脑在下。从棋上看,似乎布局方面还是弱项。如果拆开看,布局也就是国内业余高手的水平。
但中盘力量和官子极其强悍,已经达到了职业选手的水平。以目前的水平,挑战李世石还是差很多,不知道他最近还能提升多少。
打谱的感觉,很像是电脑在下。从棋上看,似乎布局方面还是弱项。如果拆开看,布局也就是国内业余高手的水平。
但中盘力量和官子极其强悍,已经达到了职业选手的水平。以目前的水平,挑战李世石还是差很多,不知道他最近还能提升多少。
这种东西假以时日,更强的硬件,更优化的算法,击败人类不过是个时间问题
杨威利0607 发表于 2016-2-3 19:28
电脑什么时候能不依赖人发明一种棋类游戏才厉害,掷骰子的那种不算
发明棋类游戏算什么?电脑如果能发明一个大卖的H游戏,那才是细致入微的厉害。
电脑什么时候能不依赖人发明一种棋类游戏才厉害,掷骰子的那种不算
发明棋类游戏算什么?电脑如果能发明一个大卖的H游戏,那才是细致入微的厉害。
AI在围棋上战胜人类只是时间问题,但三月份和老李的战局,我还是想在老李的身上压五毛钱
